What to measure?
The question of what makes a text “good” is probably as old as narrative itself and a highly complex challenge of quantitative literature studies. The complexity and heterogeneity of narrative texts, what distinguishes them from other types of texts, and what distinguishes reading narrative from different kinds of reading – but also the myriad of types of readers and possible perspectives of what is “good” in literature – ultimately complicates the issue. The question of literary quality is complex in – at least – two regards: the number of features of a text we might try to measure and the number of potential “judges” we might interrogate. Even if we can surely see that some books are more popular than others (see the most rated books on GoodReads, or the least), and even if recent quantitative studies of literature find that readers’ judgments converge at the scale of large numbers – the reasons why a book is more loved are elusive.
Large-scale studies of ”literary quality” often look at various textual features of literary works to predict, for example, reader appreciation. Yet, this is a complex endeavour. What features do we choose? What features are we overlooking? Do stylistic elements of a book, such as sentence length, the size and difficulty of their vocabulary, etc., matter more than, for example, narrative features, like which topics a book touches on or the shape of the sentiment arc of a narrative? Moreover, some features intrinsic to texts (and literary texts especially) are difficult or virtually impossible to grasp by computational means (such as metaphors or imagery).
Because they are easy to compute, stylistic features are often more explored than other types of components in quantitative studies, with various studies including some form of stylistic complexity (Maharjan et al. 2017; van Cranenburgh and Bod 2017; Bizzoni et al. 2023), using simple features or combinations of features, such as via readability formulas, to assess the “readability” of a text. Similarly, rules and recommendations of writing often center on writing style, whether we look to the author’s writing advice (such as Stephen King’s admonition on using “-ly” adverbs), to writing schools, literary scholars, publishers, or from industry, where various applications offer help with writing, such as the Hemingway or Marlowe apps. However, other forms of narrative advice have been with us for centuries. It suffices to open up Aristotle to find that a narrative should be an organic “whole” and have a beginning, middle, and end, with an orderly arrangement of parts. “Shapes of stories” may vary; perhaps some are more popular than others.
One line of inquiry of quantitative literary studies has been investigating these questions. We in the FabulaNET team have been especially exploring ways to measure story arcs’ dynamics. We have put recent effort into testing how effective sentiment arc dynamics are for modelling reader appreciation compared to other features, be they stylistic or semantic. We presented two papers at the WNU and WASSA workshop at ACL in Toronto this summer, which deep-dived into this topic, testing different feature sets against each other.
What to predict?
As noted, one thing is deciding what type of features we should test for predicting reader appreciation; another and perhaps more pressing concern is what “reader appreciation” we are trying to predict. Even if we try to put aside factors that may influence reader judgments – such as publicity or author-gender (Wang et al. 2019; Lassen et al. 2022) – we are still left with various types of literary judgments originating from different audiences: from the assessments of committees for literary prizes to GoodReads users’ ratings, to what books sell better or are more often assigned on reading lists and syllabi: it’s a rare book that is placed at the top by all of these types of readers. For our two studies, we used GoodReads online user ratings. While the GoodReads rating system has limitations, such as conflating various forms of appreciation to one scale and potential genre biases, it simplifies complex analysis. It provides a practical starting point for identifying trends across diverse books, genres, and authors. With over 90 million users, Goodreads offers valuable insight into a diverse pool of readers with varying backgrounds, genders, ages, native languages, and reading preferences.
Two experiments
To examine the weight of different features in predicting reader appreciation, we conducted experiments on the Chicago corpus of ca. 9,000 novels spanning various genres and published from 1880 to 2000, mainly written by US authors. As we were particularly interested in the combinations of features that can more accurately predict ratings, we used both a stacked ensemble model featuring a Support Vector Machine-based regressor as well as relatively simple and interpretable regression models, using a small set of “classic” models such as Linear Regression, Lasso and Bayesian Ridge – also because our interest in identifying combinations of features that can accurately predict ratings goes beyond simply achieving high accuracy. For one paper, we tested how much adding sentiment features to a stylistic feature set helped models predict GoodReads ratings of books. Our sentiment features were more or less complex, either indicating, for example, the mean sentiment of a text (how positive/negative the overall valence is) or measuring dynamics of their sentiment arcs, using approximate entropy or the Hurst exponent to gauge these dynamics. For the other paper, we compared sentiment features to semantic features, specifically topic categories from Roget’s thesaurus. Studies suggest that what and how many topics a text touches upon matters for reader appreciation to model topic distributions or “semantic profiles” of texts, lexica like LIWC (Luoto and van Cranenburgh 2021; Naber and Boot 2019) or Roget’s thesaurus have been used (Jannatus Saba et al. 2021).
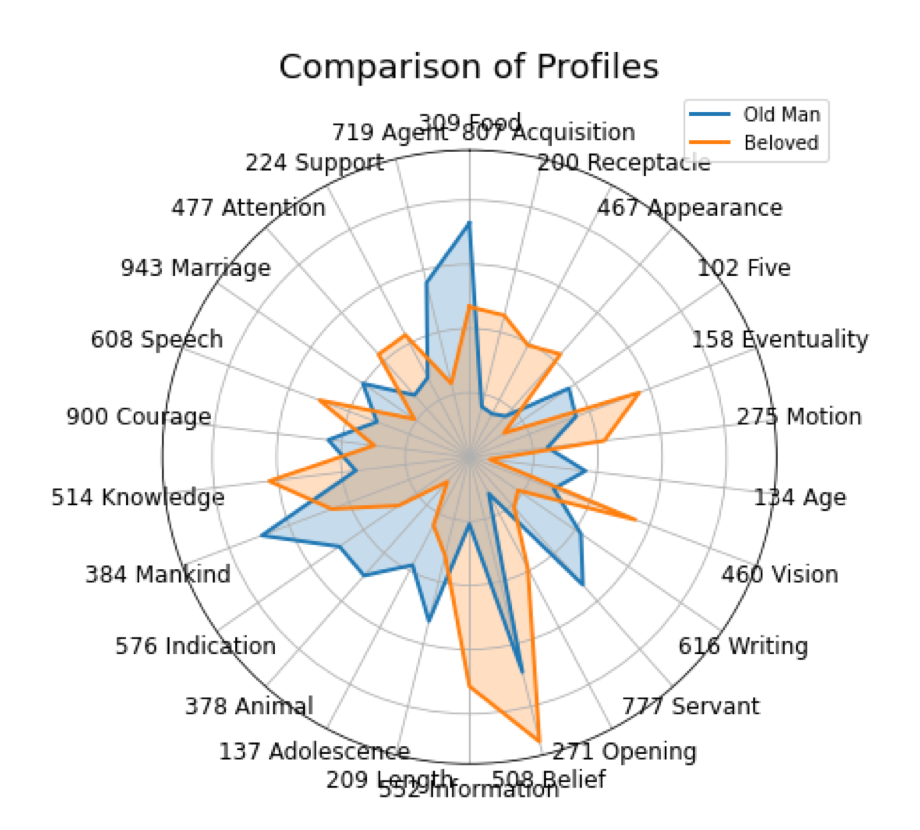
In our first paper, we showed that adding several sentiment-related features improves the predictive power of most models. We also found that the bottom 2% of titles elicited distinctly lower ratings and that their appreciation was partly predictable through the textual features we used. Finally, we analyzed the features needed to predict perceived literary quality, noting that a balance between simplicity and diversity characterizes more appreciated titles. In our second paper, we found that combining sentiment arc features, including dynamic measures, with semantic profiling based on Roget categories significantly boosts the predictive ability of regression models. In other words, considering a diverse set of psycho-semantic features (Roget categories) alongside sentiment arc dynamics outperforms using either of these methods alone. By combining these two dimensions — semantic content and sentimental dynamics — we can delve deeper into the complex interplay between emotional patterns and thematic elements that impact the perception of literary quality. Interestingly, we also found (as in the first paper) that the lowest-rated books exhibit a distinct predictability, making them stand out.
Our plans include expanding our dataset with even more texts and features. Combining various types of features, from stylometric and syntactic to semantic and sentiment features could offer deeper insights into the intricacies of literary quality perception. We also aim to explore genre-specific patterns to uncover unique insights specific to different types of literature. Additionally, we intend to move beyond GoodReads and explore alternative sources such as anthologies, awards, and canon lists, which provide more diverse and sophisticated metrics for assessing reader appreciation. This broader set of indicators will help us better understand the multifaceted factors influencing how literature is perceived.
The hard-to-predict
Our second paper found some works that models seemed to struggle with based on the chosen feature sets (sentiment and semantic features). These were famous and infamous novels like Ayn Rand’s Atlas Shrugged, William Gibson’s Neuromancer, James Joyce’s Ulysses, and Isaac Asimov’s I, Robot. One possible reason these works were complex to predict is their devoted fan base. Since the model relies on text-based features only, it can’t capture the cult-like admiration for works that are either stylistically complex (like Ulysses), less literary (like Atlas Shrugged), or straightforward (like I, Robot). These works’ status as cultural icons could influence how users rate them, going beyond the text itself.
In a more recent paper building on those outlined here, we sought to include even more features and explore these “easy” or “hard-to-predict” books further. This study compared stylistic and syntactic features to semantic features and features modeling sentiment arcs’ dynamics, looking at which books were more easily predictable based on models trained on the former or latter feature sets. Here, we found that while our models are better at predicting each their kind of books – one predicting genre-fiction better, and the other predicting stylistically outstanding works better (such as modernist prose) – they converged to find the same books hard to predict. The study, presented at RANLP 2023 can be read here (pp. 739-747).
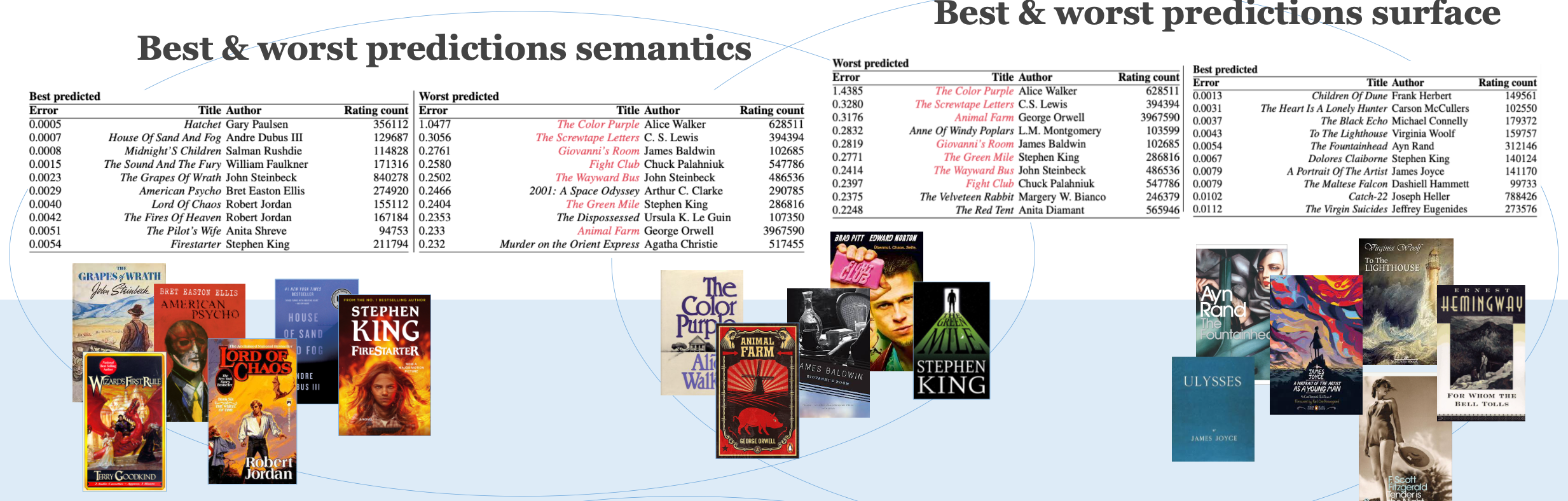
Our papers for the ACL workshops can be read here and here.
Our papers for the ACL workshops can be read here and here.
References:
Bizzoni, Y., Feldkamp Moreira, P., Dwenger, N., Lassen, I.M., Rosendahl Thomsen, M., and Nielbo, K. (2023). Good Reads and Easy Novels: Readability and Literary Quality in a Corpus of US-Published Fiction. In Proceedings of the 24th Nordic Conference on Computational Linguistics (NoDaLiDa), 42–51. Tórshavn, Faroe Islands: University of Tartu Library. https://aclanthology.org/2023.nodalida-1.5.
Cranenburgh, A. van, and Bod, R. (2017). A Data-Oriented Model of Literary Language. In Proceedings of the 15th Conference of the European Chapter of the Association for Computational Linguistics: Volume 1, Long Papers, 1228–38. Valencia, Spain: Association for Computational Linguistics. https://aclanthology.org/E17-1115.
Jannatus, S., Bijoy, S., Gorelick, H., Ismail, S., Islam, S., and Amin, M. (2021). A Study on Using Semantic Word Associations to Predict the Success of a Novel.” In Proceedings of *SEM 2021: The Tenth Joint Conference on Lexical and Computational Semantics, 38–51. Online: Association for Computational Linguistics. https://doi.org/10.18653/v1/2021.starsem-1.4.
Lassen, I.M., Bizzoni, Y., Peura, T., Rosendahl Thomsen, M., and Nielbo, K.L. (2022). Reviewer Preferences and Gender Disparities in Aesthetic Judgments. In CEUR Workshop Proceedings, 280–90. Antwerp, Belgium. https://ceur-ws.org/Vol-3290/short_paper1885.pdf.
Luoto, S., and Cranenburgh, A. van. (2021). Psycholinguistic Dataset on Language Use in 1145 Novels Published in English and Dutch.” Data in Brief 34: 106655. https://doi.org/10.1016/j.dib.2020.106655.
Maharjan, S., Arevalo, J., Montes, M., González, F. A., and Solorio, T. (2017). A Multi-Task Approach to Predict Likability of Books. In Proceedings of the 15th Conference of the European Chapter of the Association for Computational Linguistics: Volume 1, Long Papers, 1217–27. Valencia, Spain: Association for Computational Linguistics. https://aclanthology.org/E17-1114.
Naber, F., and Boot, P. (2019). Exploring the Features of Naturalist Prose Using LIWC in Nederlab. Journal of Dutch Literature 10 (1). https://www.journalofdutchliterature.org/index.php/jdl/article/view/183.
Wang, X., Yucesoy, B., Varol, O., Eliassi-Rad, T., and Barabási, A. (2019). Success in Books: Predicting Book Sales before Publication. EPJ Data Science 8 (1): 31. https://doi.org/10.1140/epjds/s13688-019-0208-6.