‘Ay’, he said aloud. There is no translation for this word and perhaps it is just a noise such as a man might make, involuntarily, feeling the nail go through his hands and into the wood.
While this sentence from Ernest Hemingway’s The Old Man and the Sea is descriptive and subtle, it may evoke strong affect for readers and give way to a range of interpretations and emotions that are difficult to quantify accurately. Recently, the interest in adequately gauging the sentiments of literary texts has gained more attention, coming out of the stronger focus on affect in literary theory and the turn to computational methods in literary analysis. Yet an open question is how adequate tools – often imported from social media analysis – work for the more subtle and descriptive form of emotional communication in literary texts.
In one of our recent studies, “Comparing Transformer and Dictionary-based Sentiment Models for Literary Texts: Hemingway as a Case-study” (Bizzoni & Feldkamp, 2023), we set out to assess the adequacy of such tools. We compare the efficacy of various computational Sentiment Analysis (SA) methods – from traditional dictionary-based approaches to Transformer models – in capturing literary texts’ emotional intensity and sentiment arcs, using Hemingway’s novel as a case study.
Why Hemingway?
Besides being a highly admired writer, Hemingway is renowned for his stylistic subtlety and his “iceberg” style, which attempts to leave as much as possible unsaid (known to the author, but not put on the page): a delicate system that results in a tense style and prompts readers to infer underlying emotions and unspoken events. By avoiding overt emotional display (Strychacz, 2002) and figurative language (Heaton, 1970), Hemingway’s minimalist approach can be particularly effective in evoking, rather than naming, a feeling or an experience. At the same time, this omissive and understated style can be highly challenging for Sentiment Analysis tools – making a Hemingway story a perfect case study for evaluating them.
How to compare annotators?
When comparing different models, it is important to establish a robust human benchmark for evaluation. However, this has its challenges, as even among human annotators, there are substantial variations in how we perceive sentiment in texts. To try to mitigate this risk, we had two annotators – both possessing literature degrees and extensive experience in literary analysis – score the valence of sentences. Their scoring provides a baseline against which we could evaluate the models’ performance. Subsequently, we compared these human annotations with the scores generated by four state-of-the-art (SOTA) Transformer models (Sanh et al., 2020; Peirsman, 2020; Barbieri et al., 2020; Barbieri et al., 2022) and two dictionary-based models (Hutto and Gilbert, 2014; Jockers, 2014). Using the average human annotator score as our reference point, we assessed how closely each model’s scores aligned with the human sentiment perception.
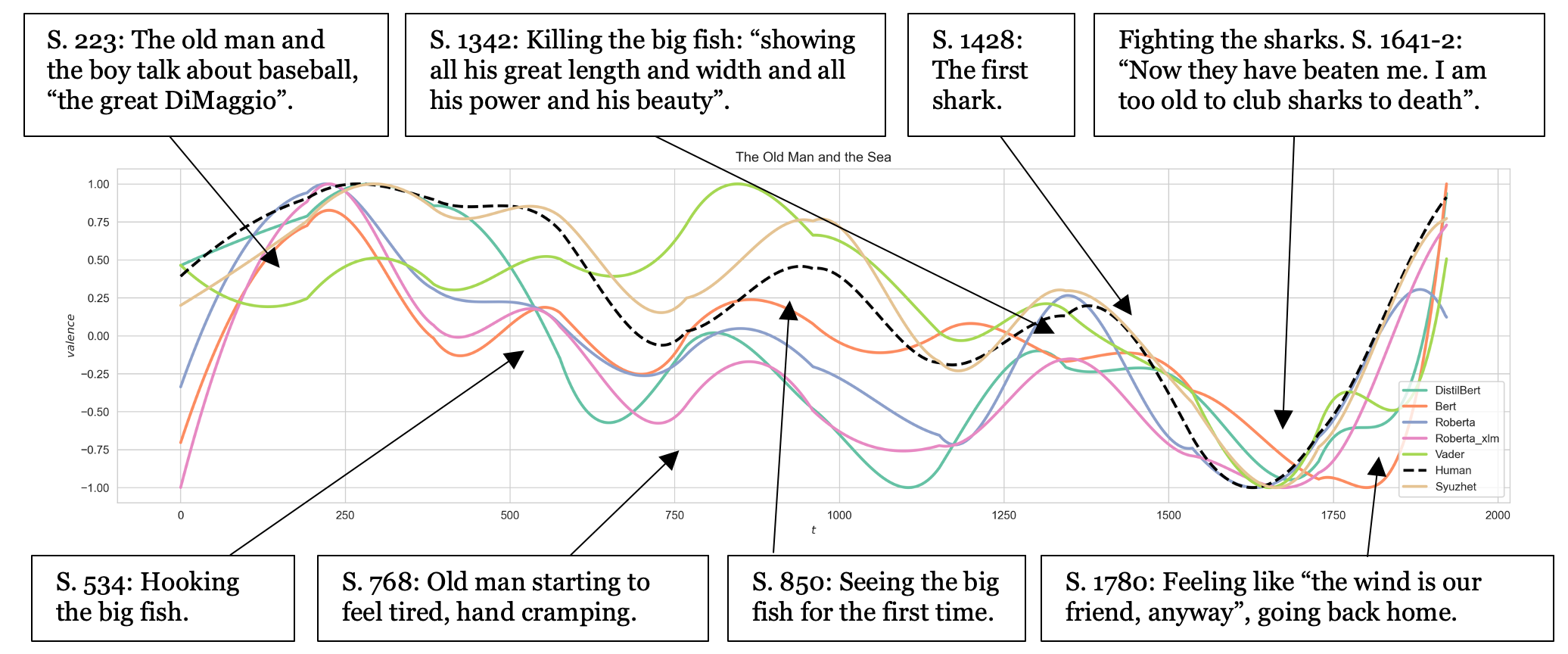
The Old Man and the Sea annotated from beginning to end with different Sentiment Analysis methods. The black dashed line is the human mean score.
Who does the better job?
The question of which method does a better job doesn’t have a simple answer. The figure visually represents this complexity, using different methods to show the narrative arc of The Old Man and the Sea. Each method’s arc deviates from the dashed line, representing the average (mean) value of human annotations for narrative events. By comparing the arcs of different methods to the dashed line, we can see how closely each method aligns with human annotations. More significant deviations from the dashed line indicate differences between the model’s output and human perception. Smaller deviations suggest better alignment with human understanding.
Our findings point in two directions. First, our research revealed that Transformer-based models generally outperformed dictionary-based approaches in correlating with human judgments on sentence-level valence. However, when analyzing detrended sentiment arcs—capturing the overall sentiment progression of a text—the dictionary-based Syuzhet model (Jockers, 2014) exhibits closer alignment with human annotations. While the Syuzhet dictionary is relatively simple, it has been constructed from human ratings of words in literary texts, an advantage we seem to see the effect of here.
In sum, we have found that different SA methods are good at different scales, underscoring the importance of selecting the appropriate tool for specific analytical needs.
Read the full paper here: https://aclanthology.org/2023.nlp4dh-1.25.pdf
References
Francesco Barbieri, Luis Espinosa Anke, and Jose Camacho-Collados. 2022. XLM-T: Multilingual Language Models in Twitter for Sentiment Analysis and Beyond. In Proceedings of the Thirteenth Language Resources and Evaluation Conference, pages 258–266, Marseille, France. European Language Resources Association. https://aclanthology.org/2022.lrec-1.27
Francesco Barbieri, Jose Camacho-Collados, Leonardo Neves, and Luis Espinosa-Anke. 2020. TweetEval: Unified Benchmark and Comparative Evaluation for Tweet Classification. In Findings of the Association for Computational Linguistics: EMNLP 2020, pages 1644–1650, Online. Association for Computational Linguistics. https://aclanthology.org/2020.findings-emnlp.158
Yuri Bizzoni and Pascale Feldkamp. 2023. Comparing Transformer and Dictionary-based Sentiment Models for Literary Texts: Hemingway as a Case-study. In Proceedings of the Joint 3rd International Conference on Natural Language Processing for Digital Humanities and 8th International Workshop on Computational Linguistics for Uralic Languages, pages 219–228, Tokyo, Japan. Association for Computational Linguistics. https://aclanthology.org/2023.nlp4dh-1.25.pdf
C. P. Heaton. 1970. Style in The Old Man and the Sea. Style, 4(1):11–27. Penn State University Press. https://www.jstor.org/stable/42945039
Clayton Hutto and Eric Gilbert. 2014. VADER: A Parsimonious Rule-based model for Sentiment Analysis of Social Media Text. In Proceedings of the international AAAI conference on web and social media, volume 8, pages 216–225. https://doi.org/10.1609/icwsm.v8i1.14550
Matthew Jockers. 2014. A Novel Method for Detecting Plot. Matthew L. Jockers Blog. https://www.matthewjockers.net/2014/06/05/a-novel-method-for-detecting-plot/
Victor Sanh, Lysandre Debut, Julien Chaumond, and Thomas Wolf. 2020. DistilBERT, a distilled version of BERT: smaller, faster, cheaper and lighter. ArXiv:1910.01108. https://arxiv.org/abs/1910.01108
Thomas Strychacz. 2002. "The Sort of Thing you Should not Admit": Ernest Hemingway's Aesthetic of Emotional Restraint. In Milette Shamir and Jennifer Travis (Eds.), Boys Don't Cry? Rethinking Narratives of Masculinity and Emotion in the U.S., pages 141–166. Columbia University Press. https://doi.org/10.7312/sham12034-009