“It was lovely summer weather in the country, and the golden corn, the green oats, and the haystacks piled up in the meadows looked beautiful.”
With such beautiful scenery begins the fairy tale of “The Ugly Duckling”. If you are familiar with the story, you know that it will not be this happy and peaceful all the time. The plot includes a lot of twists and turns, with a surprising ending. There seems to be something compelling in this narrative. The story of a “duckling” turning out to be a beautiful swan; and its moral, not judging anyone by their outward appearance, has been loved by readers since its publication in the mid-19th century.
In the previous post, we introduced our method to apply fractal theory to computational narrative analysis. This way, we aim to model the dynamics in a narrative, and describe the dynamical structure and distribution of positive and negative sentiments throughout the whole narrative.
Matthew Jockers has already done pioneering work in the field of narratives and sentiment arcs (Jockers, 2015). Moreover, it has been suggested that there are 6 kinds of narrative archetypes (Reagan et al., 2016).
Thus, our method builds upon these findings. Rather than looking at what kind of narrative arc a story has, we want to know why they are such, and why readers enjoy such arcs. As we discussed in the previous post, the sentiment dynamics in a story can be computationally modeled through the Hurst exponent which measures self-repetition in the sentiment structure at different time scales. We proposed that an optimal value for Hurst concerning the sentiments would be between 0.55 and 0.65. Now, we aim to prove that our hypothesis is actually supported by experimental findings.
To do this, we need to measure the sentiment dynamics and compare it with the actual reader appreciation of the story. As a first case study, we wanted to apply our methods on a corpus that we know well, to evaluate that the computed sentiment arcs actually capture the underlying narrative dynamics in a story and, consequently, that we can trust our findings.
As Fabula-NET is based in Aarhus, Denmark, what could be a better exploration than selecting a Danish author, Hans Christian Andersen, whose fairy tales have been read and loved worldwide?
First, the fairy tales are relatively short and have simple narrative structures: we know the sentiments that the sentiment arc should capture – the little match seller gets more and more sad, while the ugly duckling has a happy ending. This allowed us to qualitatively check and interpret the sentiment arcs. Second, Andersen’s tales are widely read in English, and we could use the state-of-the-art sentiment tools to compute our arcs. Finally, as the whole corpus consists of texts written by one author, falling within one genre with a relatively uniform style, we could control for related, undesired variables affecting the current analysis and results.
Reading with a sentiment lexicon
Of the available sentiment tools we decided on using the NRC-VAD lexicon by Said Mohammad (Mohammad & Turney, 2013). It is composed of almost 20.000 English words that are annotated for sentiment. We retrieved the scores for all words that could be found in the lexicon, and assigned a neutral value to others. The example illustrates how most of the missing words are small function words or pronouns that we do not expect to have much sentiment value.
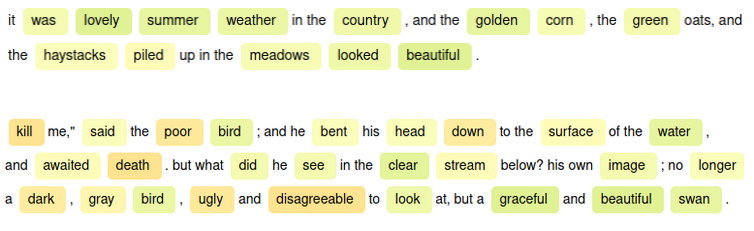
Two extracts from The Ugly Duckling, “read” with the sentiment lexicon, the first sentence and the turning point towards the end. The greener the word is, the more positive score it gets in the lexicon.
While this word-by-word method does not account for negations or context, it allows us to be very transparent. We rely on the assumption that each word has intrinsic associations to sentiments, regardless of the context. The adjective “ugly” would have negative connotations regardless of its relative position in the happy ending of a story. Moreover, the sentiment arcs were smoothed using adaptive fractal analysis which fits the sentiment arc with the best polynomial order found using the standard least-squares regression (Gao et al., 2011). Below, you can see an example of the raw sentiment arc and the polynomial fits that amplifies the sentiment trends from the noise. In a way, the raw signal, our ugly duckling, develops into a sentimental ride that the reader experiences along the story.
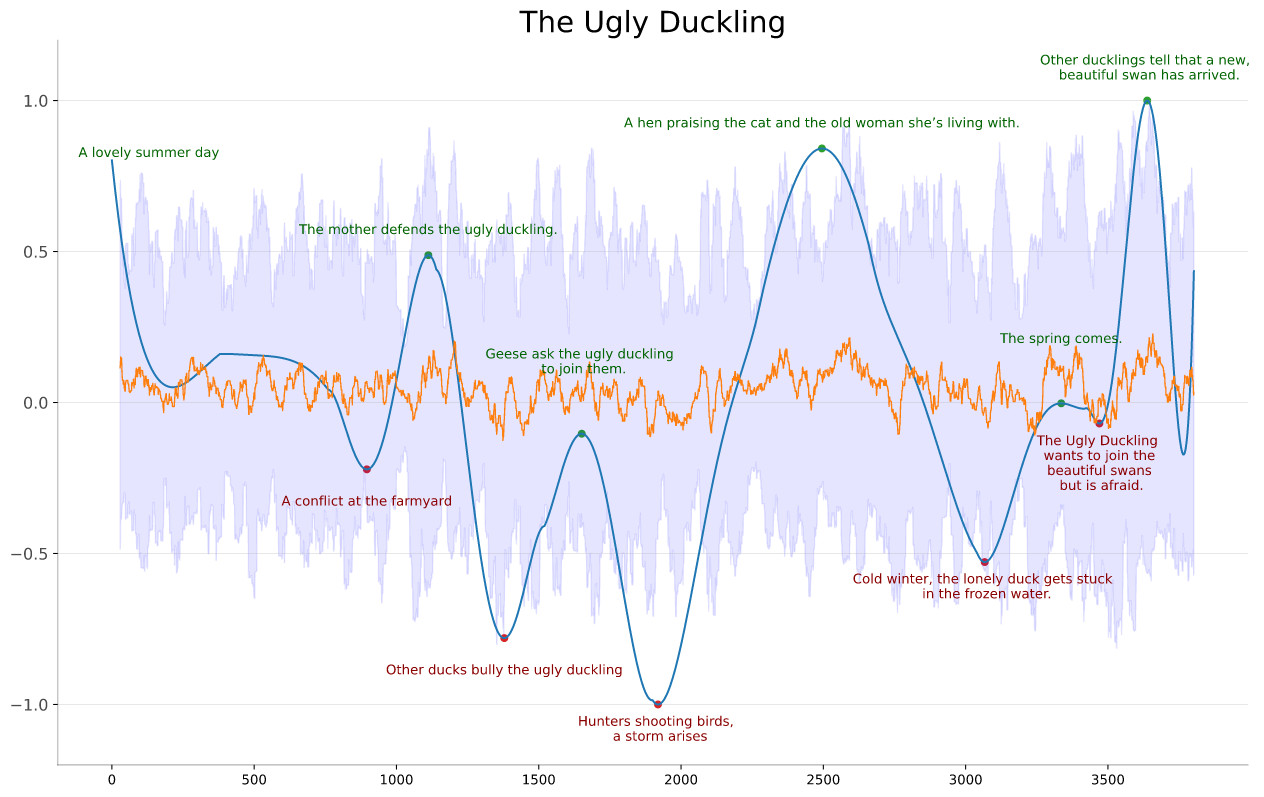
The raw signal (in orange) and the smoothed sentiment arc (in blue), with the most important narrative peaks and troughs in the Ugly Duckling.
What do readers think about fractality?
Once we had obtained the Hurst values for all H. C. Andersen tales in the corpus, we still needed to correlate these values to the opinions of readers. Would they prefer the stories having a Hurst between 0.55-0.65, as we expected?
To obtain a large, crowd-sourced pool of human annotations, we resorted to GoodReads, which is a popular, social platform for readers to grade, discuss and recommend books. We used the GoodReads grades to approximate the perceived quality of the fairy tales: the higher the average score and the more ratings, the more beloved a story is. For instance, the ugly duckling has got an average score of 4.10/5 points, and the number of raters is 40,750.
The next step was to carry out correlation analyses between these two scores: the Hurst exponent and the GoodReads rating. We find that there is a positive correlation between the scores and the ratings. Stories that are more popular tend to cluster towards the higher end, whereas less known and liked stories are at the lower end. It is also worth noticing that most stories fall between H=.54 and H=.58.
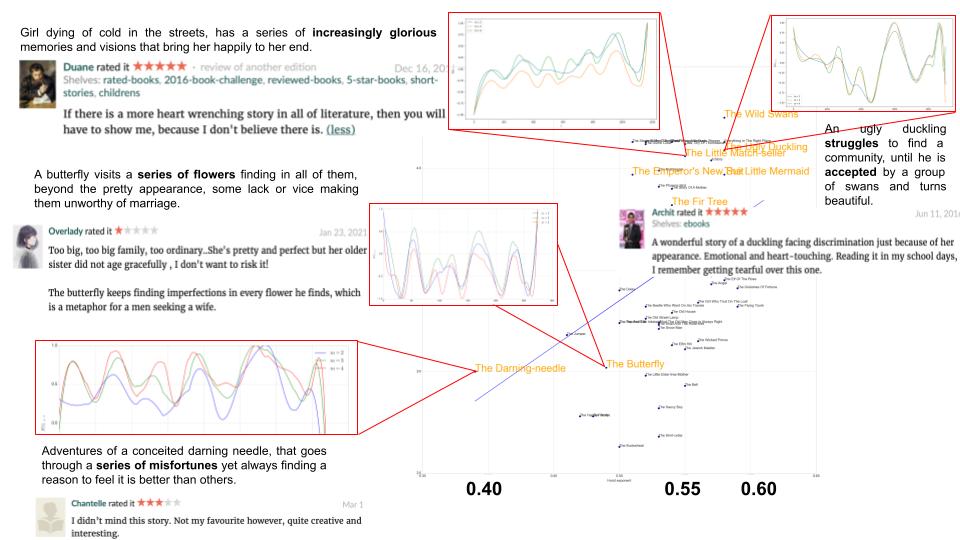
A visual summary of our method. Hurst values (x-axis) are correlated with the GoodReads scores (y-axis), illustrated with some comments from the GoodReads users. Fairy tales that have a too simple, repetitive structure, such as The Butterfly, receive a lower rating in GoodReads. Stories with a more complex sentiment structure also receive a higher score from the readers.
Thus, there is something H. C. Andersen does intuitively to build a sentiment arc in a story, and what readers seem to appreciate.
It is interesting to see that there is a correlation between the sentimental coherence and the perceived quality of a fairy tale. This encourages further use of multifractal theory in the study of narratives. Of course, there are many other components that lead to literary appreciation, which now looks like noise in the signal. Now that we proved the method works on a gold-standard fairy tale corpus, we can continue exploration with a bigger corpus of texts to confirm these preliminary tendences. While it was successful with one Hurst value, we might want to fit that more dynamically (Hu et al., 2021), and it would be interesting to move on to sentence-level sentiments or even to emotion analysis instead of the lexicon-based approach, to get a more contextual look of sentiments.
Furthermore, the current focus on sentiment arcs ignores other dimensions of a text, such as linguistic features, word concreteness, and sentence-level information to obtain a more informed insight into what leads to literary appreciation. Indeed, a sweet spot of structural predictability and interesting variation encompasses all these components.
In this sense, the present findings are one successful step in the story: we have detected a persistent signal in this interplay of components, suggesting that fractal patterns, previously identified in other cultural phenomena (Gao et al., 2012) are also found in story narratives through, at least, their sentimental dimension.
References:
Gao, J., Hu, J., Mao, X., & Perc, M. (2012). Culturomics meets random fractal theory: Insights into long-range correlations of social and natural phenomena over the past two centuries. Journal of The Royal Society Interface, 9(73), 1956–1964. https://doi.org/10.1098/rsif.2011.0846
Gao, J., Hu, J., & Tung, W. (2011). Facilitating joint chaos and fractal analysis of biosignals through nonlinear adaptive filtering. PloS One, 6(9), e24331.
Hu, Q., Liu, B., Gao, J., Nielbo, K. L., & Thomsen, M. R. (2021). Fractal scaling laws for the dynamic evolution of sentiments in Never Let Me Go and their implications for writing, adaptation and reading of novels. World Wide Web, 24(4), 1147–1164. https://doi.org/10.1007/s11280-021-00892-5
Jockers, M. (2015). » Revealing Sentiment and Plot Arcs with the Syuzhet Package from https://www.matthewjockers.net/2015/02/02/syuzhet/
Mohammad, S. M., & Turney, P. D. (2013). Nrc emotion lexicon. National Research Council, Canada, 2.
Reagan, A. J., Mitchell, L., Kiley, D., Danforth, C. M., & Dodds, P. S. (2016). The emotional arcs of stories are dominated by six basic shapes. EPJ Data Science, 5(1), 1–12.