Biterm Topic Model#
The tweetopic.BTM class provides utilities for fitting and using Biterm Topic Models
The Biterm Topic Model is a generative probabilistic model for topic modelling. Instead of describing the document generation process, biterm models focus on describing how word coocurrances are generated from a topic distribution. This allows them to capture word-to-word relations better in short texts, but unlike DMM, they can also work well for corpora containing longer texts.
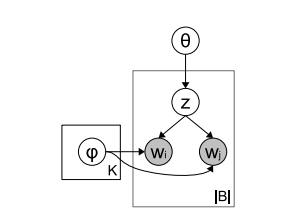
Graphical model of BTM with plate notation (Yan et al. 2013)
BTMs in tweetopic are fitted with Gibbs sampling . Since Gibbs sampling is an iterative ḾCMC method, increasing the number of iterations will usually result in better convergence.
Usage#
Creating a model:
from tweetopic import BTM
btm = BTM(
n_components=15,
n_iterations=200,
alpha=6.0,
beta=0.2,
)
Fitting the model on a document-term matrix:
btm.fit(doc_term_matrix)
Predicting cluster labels for unseen documents:
btm.transform(new_docs)
References#
Yan, X., Guo, J., Lan, Y., & Cheng, X. (2013). A Biterm Topic Model for Short Texts. Proceedings of the 22nd International Conference on World Wide Web, 1445–1456. Παρουσιάστηκε στο Rio de Janeiro, Brazil. doi:10.1145/2488388.2488514